Innovation in Drug Discovery: How the Disease Model Finder Is Changing the (Oncology Research) Game
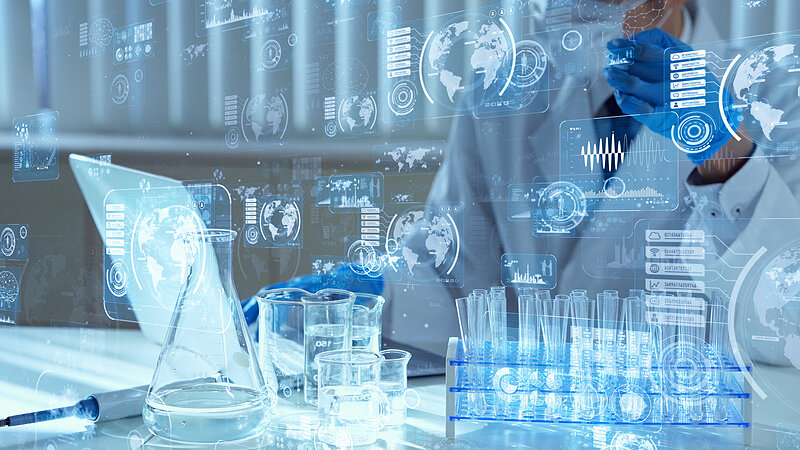
The field of preclinical oncology research is being hit by a new wave of innovation, specifically innovation in disease model sourcing for drug discovery. In this article, we teamed up with Javier Pineda, PhD, data scientist here at Scientist.com, for a Q&A surrounding the challenges associated with sourcing disease models and how these can be overcome by leveraging the power of machine learning.
How has the landscape for preclinical oncology research changed in recent years?
I would say oncology research has been changed by the constant search for more physiologically relevant models – the closer to human physiology, the more credible the research and its findings. For this reason, mouse models have been an integral part of cancer drug discovery. However, now that patient derived xenografts have entered into the scene, there has been more of an approach toward personalized medicine. Now, we can take human tumor biopsies and implant them into mice to test for the most appropriate therapeutic intervention. The main component missing here, of course, is a functional human immune system, and it is this point that has brought about a demand for more humanized mouse models, which can better recapitulate the situation observed in cancer patients.
Why is it important to have an appropriate model for drug discovery projects, and what happens when the model is not right?
Using the wrong disease model can be costly for a drug discovery project. For example, many drug candidates that appear to be active in cell culture models are later found to be inactive in animal models, or worse, they are found to be inactive in clinical trials. In many cases, the efficacy of a drug is highly dependent on a specific set of mutations or an upregulated biological pathway, which may be altered depending on the model used. The models themselves can be expensive to source, as in the case of patient-derived xenografts, so it’s critical that all these specifics are taken into account for the overall success of a research study.
Can you provide an overview of the type of models that pharma and biotech researchers have to usually sift through for their projects?
Sure, so there are several types of models that researchers may use for their projects. There are immortalized cell lines, primary cell lines derived from patient tissue, 3-D models such as spheroids or organoids. In the realm of oncology, there are also syngeneic mouse models and, of course, xenografts.
Please elaborate on what a patient-derived xenograft (PDX) model entails.
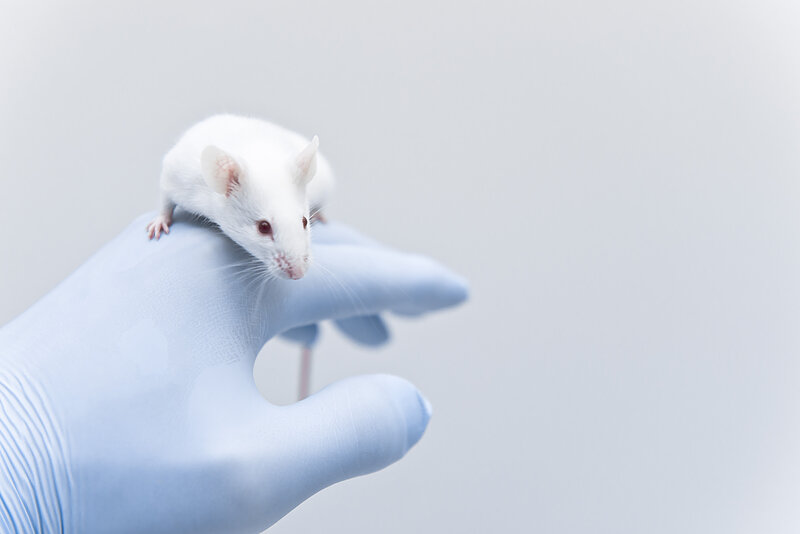
Patient-derived xenografts involve the implantation of human patient cancer tissue into a mouse. Typically, this requires the use of an immunocompromised mouse so that the human cancer tissue is not rejected by the mouse’s immune system, though these days it is also possible to use humanized mice instead, which have reconstituted human immune systems.
What are the current challenges associated with sourcing disease models?
The first and biggest challenge that many researchers face is where they can find the disease models that pertain to their studies. What we have found in working with researchers is that many of them need to apply a “shotgun” approach to finding the right supplier; they will reach out to suppliers they are familiar with, ask about model availability, and cross their fingers that at least one of those suppliers has what they need. The second challenge, of course, is negotiating a project proposal and taking care of any necessary paperwork, which for some companies can take several weeks to months.
What sort of data is already available for aiding researchers in model selection? What is lacking?
In general, researchers can access most relevant annotation-level data about disease models from supplier websites and catalogs as well as a few third-party sites. This gives them access to information such as disease type and subtype, mouse strains used, tissue culture passage, geophysical location of the disease model, as well as basic patient information.
The problem is that such annotation-level data is often not enough for researchers who also need to look into genetic mutations, expression of target genes, or drug sensitivity before sourcing a disease model. Researchers may be able to obtain some molecular data from a supplier, but because of the proprietary nature of the data, these researchers cannot readily make quantitative comparisons between models provided by different suppliers.
How can these challenges be solved?
Scientist.com has aimed to solve all of these challenges. Our research concierge team is always ready to suggest suppliers who are more likely to have the disease model that a researcher requires. Also, the Scientist.com platform also takes care of the paperwork issue and drastically shortens the time between an initial request and project initiation.
That being said, there are still thousands of disease models that researchers may require for their projects, and most researchers wish that they could see all relevant models in one database and ideally obtain molecular data across all suppliers; that way, the researchers themselves can make quantitative comparisons and a final informed decision about the models they wish to source.
This is one of the core challenges experienced by researchers that we have aimed to solve with the Disease Model Finder. With the relationship that Scientist.com has with many disease model suppliers, we have a unique position that allows us to aggregate data across suppliers, ensure data confidentiality and security, and enable researchers to make quantitative comparisons between disease models with respect to genomic and transcriptomic data.
What does the Scientist.com Disease Model Finder enable your customers to achieve that they couldn’t before?
In addition to enabling researchers to make quantitative model comparisons across suppliers, the Disease Model Finder also works in concert with the COMPLi platform at Scientist.com to guarantee supplier compliance and allows for automated purchasing through the Scientist.com marketplace. As soon as the researcher finds the model they need, they can purchase the model or request services immediately.
The Disease Model Finder provides many details pertaining to each disease model, but it also provides insight into where each model falls in relation to the others.
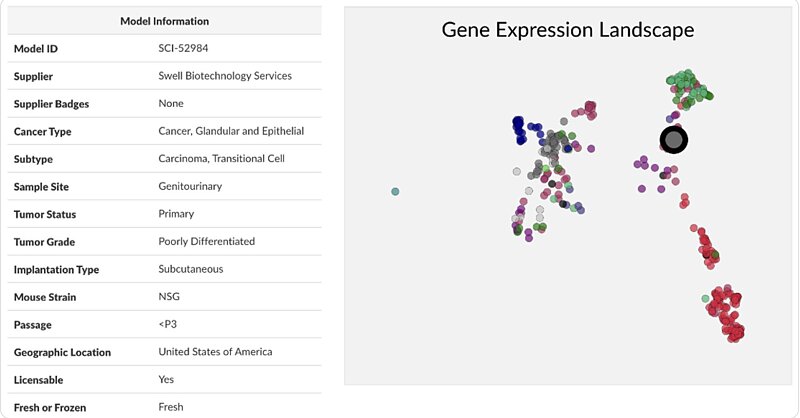
How do machine learning algorithms help filter and sift through data and how do they help in appropriate model selection?
The Disease Model Finder works with large, molecular, sequencing-based datasets from multiple suppliers, and this is a task that demands proper data handling. For example, to allow researchers to compare models across these suppliers, the Disease Model Finder makes use of several machine learning algorithms, such as clustering algorithms and dimensionality reduction algorithms, to process and aggregate the data. These algorithms also enable researchers to visualize high-dimensionality datasets in 2-D for direct, visual model comparisons, as well as conduct differential gene expression analysis for models of interest. In combination with the multifaceted filter functionality, this allows researchers to find and source appropriate models for their research much more efficiently.
What insights do you think the Disease Model Finder can provide? (model types, fresh/frozen sample types, gene types)
The Disease Model Finder provides many details pertaining to each disease model, but it also provides insight into where each model falls in relation to the others. For example, a researcher will be able to determine whether a specific colon cancer model is representative of colon cancer in general, or whether the model is more biologically distinct due to the presence of gene mutations or differentially expressed genes. Our comparison functionality can also help researchers find models that may be biologically similar across suppliers, whether in relation to proprietary gene sets or conventional pathways. This can be especially useful when conducting a large study that demands a panel of disease models.
How did the collaboration to make this resource originate?
We came up with the idea for the Disease Model Finder after having many conversations with researchers who use our platform. They had experienced much of the same issues I mentioned earlier, especially with having to contact multiple suppliers for a single study and having trouble obtaining the data necessary to QC or compare models. That was enough for us to start designing the blueprint for the Disease Model Finder, which was fine-tuned as we continued to reach out to researchers and suppliers about a more centralized and data-driven approach to disease model sourcing at Scientist.com.
What is your vision for the future of this work and the oncology field?
We are constantly striving to improve the utility of this application. That being said, we have plans to expand the scope of the Disease Model Finder from PDX models to all oncology models in the coming months. We also aim to incorporate additional bioinformatic tools that will provide researchers with disease model recommendations based on their search parameters. The platform at Scientist.com is constantly evolving to the needs of our clients, and the Disease Model Finder is a perfect manifestation of that.
Could you provide us your concluding thoughts on how cross-functional sector interaction between AI and biotech is expected to evolve in the future?
One thing I anticipate seeing more and more of in oncology research (and disease research in general) is AI-based drug-response predictions. Predicting whether a patient will respond to a therapeutic modality is a critical need in the oncology field, but it can be very difficult to make such predictions since they require a large pre-existing set of drug response data, as well as several other types of molecular data. Before even speaking about patients, most PDX models have only been tested against a few standards of care, if any. Cancer model sourcing would be tremendously improved with sufficient predictions into drug response, but this is an undertaking that will likely improve with time.